The technology that can reduce EV range anxiety
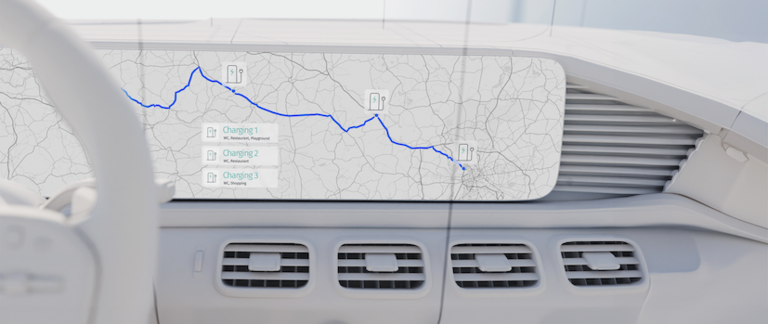
Fear of running out of power is still what holds many potential electric vehicle (EV) owners back. The right data combined with machine learning can help.
2030 might seem a long way away, but the automotive industry is already planning for it. To meet zero emissions targets and demand from consumers, a significant proportion of vehicles that will be sold overall will be electric.
Some experts estimate there will be as many as 26 million electric vehicles sold by 2030. When you compare that with the 6.6 million sold in 2021, you can see that the projected growth is significant.
“We're already approaching the landmark where 10% of all vehicles sold are EVs," said Chris Handley, VP and Business Unit Head, HERE.
However, tailwinds including the increased price of gas in the past year and mandates to reduce the number of internal combustion engine (ICE) vehicles on the roads are not the only forces at play.
Obstacles still remain if ambitious targets are to be met. Not least among them is the need for further charging infrastructure, and the uncertainty many consumers still face about how and when to charge their EV.

Huge investment in charging infrastructure is needed to meet demand.
Machine learning for accurate forecasts
As electric vehicle adoption rates improve and prices go down, users are less likely to have a private charging point. This makes the need for charging stations more critical than it was when EV ownership was the preserve of the wealthy few.
“Governments are now offering tax credits and loans to build the infrastructure to meet that demand," said Handley.
Nevertheless, a significant shortfall remains. Even with predicted improvements to battery technology, drivers need solutions that help them with planning. Another major factor is that many charge points are out of service. In the US, the figure can be as high as 20% at any one time. Added to that, EV range can vary according to the temperature, wind, road surface and other aspects.
Read more — There aren't enough EV charging stations in the US. Here's why you should still electrify your fleet
To forecast range accurately, predictive data sets are required. These depend on complex models that use machine learning to calculate better ETAs. Included in the data sets are the basic routing data (maps and historic traffic information), in-vehicle data such as vehicle type and battery performance, along with information including charge point availability and charge network membership.
These inputs will eventually be added to other contextual data that can provide even further precision, including driving style, past decisions by the driver and heating, ventilation and air conditioning (HVAC) information.
Speaking on the recent HERE webinar, Solving EV Challenges with Predictive Machine Learning, VP and Business Unit Head at HERE Chris Handley explained:
“When we as an industry first began creating basic routing equations, we started with just a map, and figuring out what the shortest distance was. Then we overlaid traffic conditions or historical traffic conditions to derive ETAs.
“But when we get to EV vehicle range, which is an important factor in determining a route, we now have to look at multiple factors."
Both internal vehicle data and external predictive data are required to achieve reliable range accuracy. As more data continues to get added, ever greater precision will be achieved.
To enable machine learning, HERE — one of the largest consumers of probe data in the world — has compiled a historical library of previous EV journeys, and applied map conflation to them. Merging multiple maps and data sets together into one delivers greater accuracy, freshness and speed.
The system looks for correlating factors and works out what the scenario is likely to be based on this information while protecting the privacy of individual drivers. For example, we can use data on the amount of time people typically wait at specific charge points to tell us how long people are likely to have to wait in the future. If vehicles regularly stop at a charging station for a very brief time, it can be inferred that the charger is not in working order. As different datasets are added, a more precise estimation can be made about the availability of each charge point.
“All of this data sits behind a sensitive and sophisticated set of anonymization and privacy controls," Handley added.
Sign up for our newsletter
Why sign up:
- Latest offers and discounts
- Tailored content delivered weekly
- Exclusive events
- One click to unsubscribe