Privacy (and data) regained – with privacy conscious machine learning
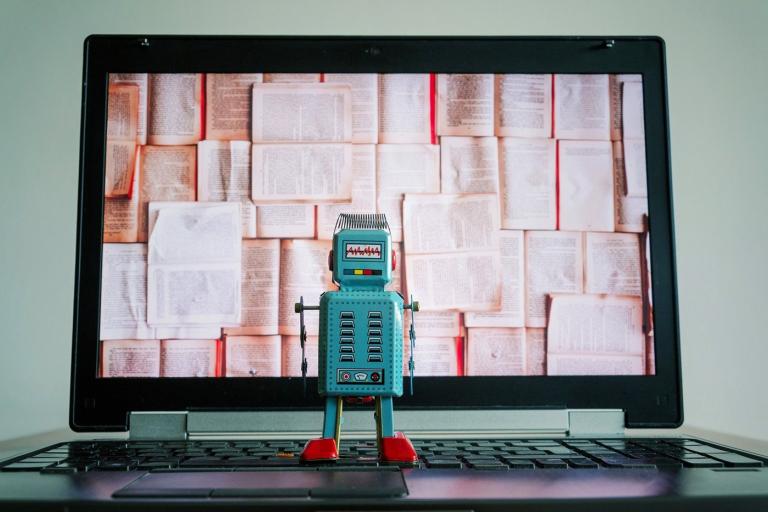
Imagine two ends of a scale. On one end, you have the sheer bulk of all the data that our devices and services collect about us. At the other end is the need for privacy at both the personal and enterprise levels. Federated learning can help balance it out.
Personally, I am very excited by the fact that HERE has a mandate to work with data and machine learning in ways that are respectful and accommodating to people’s concerns about privacy, data ownership and control of their data.
Federated learning is one potential avenue for learning from user or company data while still giving those entities a degree of control and privacy. This is because federated learning makes it possible that end users and businesses never need to transfer their data to us.
But that leaves the question, how does it even work? How can we learn from what is now unseen? Let’s compare how machine learning commonly works now to how it could work in the future.
Currently, you’re likely sending a great deal of your data directly to a company. Where you go, what you search for online, the places you eat, the places you shop, etc. All of that information goes to a data center in the cloud. The company which owns that cloud can then use your data to create new models and produce new insights. This is how the data industry makes their money right now.
As an alternative, we can instead move a model to where the data is directly collected.
In this approach, we transfer the model to you, the person or the business, to learn from your data locally. When the data is aggregated and modeled locally, only the derived knowledge, or model parameters, is shared back to the cloud, without personal information. Now, your personal data has an added layer of protection while the relevant information can be used to improve the cloud-based service.
This idea is quite powerful, and it’s easy to grasp how it could benefit smartphone users, auto owners or even drone operators as that technology evolves. These objects are among what we commonly call ‘edge sensors’, since they provide data close to the edge of the areas that we’re examining. There is another powerful application:
Companies as edge sensors
Imagine you work with multiple companies that are in the same market, like two different auto manufacturers.
Each company, A and B, has their own data sets which they collect from their vehicle fleets on the roadways. Each company has their own data center, and neither company wants to directly intermix their data. Company A doesn’t want to send their data to Company B. Company B doesn’t want to store all their data together with Company A.
Imagine if we said to each company that we can learn locally from each independent data center in a very similar way, then receive back only the general insights from that learning. We can then pool that knowledge so that both companies get better models and insights than if each used their individual data sets.
Neither company has to send any protected data or intermix it with the other company’s data, as we act as the honest broker between each. This gives a sense of how privacy-conscious distributed learning can work – or federated learning.
This is very new approach, and it can unlock a few new services into the market where privacy concerns are paramount. Medical centers can improve models to detect suspicious lesions on images without needing to share personal details of the individuals being treated. Auto manufacturers can learn to detect weather patterns or unusual vehicle behavior across thousands of vehicles.
I am excited that our field is addressing the challenge posed by the foundation of its own success: how to learn from massive amounts of data without needing to "own" the underlying data? We’ve seen some tentative moves in this direction, the blending of certain lines of attack, and this field of privacy-conscious machine learning will play an increasingly important role in a company’s data strategy.
Clearly, there is a role for HERE in this – if HERE can be this trusted intermediary via our Open Location Platform – we can help our customers unlock the value of their data, and also respect our customer’s privacy and data locality at the same time. For chess fans, it’s Privacy’s Gambit Accepted.
Michael Sprague is a Principal Data Scientist at HERE in Platform Research. He is co-author, along with colleagues Amir Jalalirad, Marco Scavuzzo, Catalin Capota, Moritz Neun, Lyman Do, and Michael Kopp, of the recently published paper “Asynchronous federated learning for geospatial applications,” which was selected as Best Paper by the Fraunhofer Institute. Bala Divakaruni, Anthony Passera, Heidi Fox, and Soojung Hong also contributed to the project. You can download a copy here
Have your say
Sign up for our newsletter
Why sign up:
- Latest offers and discounts
- Tailored content delivered weekly
- Exclusive events
- One click to unsubscribe